The Role of Machine Learning in Enhancing Agentic Commerce Experiences
- Paul Andre de Vera
- 12 minutes ago
- 6 min read
Machine learning transforms the relationship between consumers and brands by enabling intelligent, personalized interactions across digital commerce channels. Algorithms analyze purchasing patterns, preferences, and behavioral data to create customized experiences and anticipate customer needs. This shift toward agentic commerce, where AI serves as an intermediary decision-making assistant, fundamentally alters traditional retail paradigms. The technology doesn't just recommend products; it actively shapes the entire customer journey through predictive insights, creating a new frontier of commercial engagement worth exploring.
Understanding the Symbiosis of Machine Learning and Agentic Commerce
As commerce evolves in the digital landscape, machine learning and agentic commerce form a powerful partnership that transforms how consumers interact with brands and retailers.
This symbiosis enables AI agents to analyze vast datasets, uncovering nuanced customer preferences and behavioral patterns that drive personalized experiences.
Machine learning algorithms empower agentic systems to adapt continuously, making real-time adjustments based on emerging trends and changing consumer behaviors.
The relationship yields operational efficiencies through automated inventory management and price optimization while enhancing customer experience through sophisticated, learning-based interactions requiring minimal human oversight.
How LLMs Are Revolutionizing Customer Interactions in Digital Retail

Large Language Models are transforming retail by crafting personalized shopping journeys that adapt to individual preferences and past behaviors in real-time.
These AI systems leverage predictive demand intelligence to anticipate consumer needs, suggest relevant products, and optimize inventory management across digital platforms.
The integration of LLMs enables seamless multi-channel interactions where customers experience consistent service whether shopping via website, mobile app, or conversing with AI assistants.
Personalized Shopping Journeys
While traditional e-commerce platforms often struggle with generic customer experiences, Large Language Models have transformed digital retail by enabling truly personalized shopping journeys.
LLMs analyze purchase history and browsing behavior to deliver real-time personalized recommendations that adapt to seasonal trends, considerably improving conversion rates.
These AI agents synthesize information from multiple sources—public reviews, expert opinions, and individual preferences—to provide extensive, trustworthy guidance with minimal customer input.
The resulting customer engagement extends beyond transaction efficiency to foster long-term loyalty, as consumers increasingly prefer tailored experiences that anticipate their needs rather than merely responding to explicit requests.
Predictive Demand Intelligence
Beyond basic forecasting methods, predictive demand intelligence powered by LLMs represents a paradigm shift in how retailers anticipate consumer needs.
These sophisticated models analyze vast consumer behavior datasets, enabling retailers to predict shopping patterns with unprecedented accuracy.
LLMs excel at identifying complex correlations between customer preferences and market fluctuations, generating real-time insights that optimize inventory management decisions.
By forecasting demand fluctuations, retailers minimize both overstock scenarios and revenue-draining stockouts.
This intelligence extends beyond simple trend analysis, incorporating seasonal variations and individual consumer behaviors to create a dynamic supply chain that responds proactively rather than reactively to market demands.
Seamless Multi-channel Interactions
In the fragmented retail landscape, LLMs serve as the connective tissue, enabling seamless multi-channel interactions between consumers and brands. These AI systems understand and generate human-like text across digital platforms, creating cohesive customer experiences regardless of entry point.
LLMs analyze contextual consumer behavior to deliver hyper-personalized recommendations and proactive support. This intelligence allows retailers to automate complex service tasks while maintaining conversational authenticity. The technology adapts to individual preferences, creating tailored interactions that feel natural yet sophisticated.
Companies implementing these systems report significant improvements in engagement metrics and conversion rates, transforming disjointed customer journeys into unified, personalized commerce experiences.
Personalization at Scale: ML-Driven Product Recommendations
Preference prediction models form the backbone of modern e-commerce platforms. They analyze customer data to anticipate future purchasing decisions with remarkable accuracy.
These models leverage behavioral pattern analysis to identify subtle correlations between browsing habits, purchase history, and product interactions that would remain invisible to traditional analytics.
The continuous refinement of these algorithms enables retailers to present increasingly relevant product suggestions that evolve alongside consumer preferences, creating a virtuous cycle of improved customer satisfaction and higher conversion rates.
Preference Prediction Models
Preference prediction models harness the power of sophisticated algorithms and represent a cornerstone of modern e-commerce personalization strategies. These systems analyze vast datasets of customer behavior to generate hyper-personalized product recommendations that can boost conversion rates by up to 25%.
These models' real-time capabilities enable businesses to adapt recommendations dynamically based on browsing patterns and emerging trends. Beyond simple suggestions, machine learning facilitates anticipatory commerce—predicting needs before customers articulate them.
Companies implementing these systems report a 15% increase while reducing abandonment rates. The continuous learning mechanisms guarantee recommendations remain relevant, allowing businesses to maintain a competitive advantage in rapidly evolving marketplaces.
Behavioral Pattern Analysis
Modern e-commerce platforms leverage behavioral pattern analysis to transform raw customer data into actionable intelligence that drives personalized shopping experiences. By examining purchase history and browsing behavior, machine learning algorithms identify patterns at scale, delivering hyper-targeted product recommendations that increase click-through rates by 25-38%.
Advanced techniques like collaborative filtering and clustering enable businesses to anticipate consumer needs before explicitly stating them. These systems continuously refine their accuracy by learning from each interaction, adapting to evolving preferences.
The business impact is considerable—personalized recommendations greatly enhance customer retention and increase average order values as shoppers engage with relevant offers that resonate with their unique needs.
Predictive Analytics: Anticipating Customer Needs Before They Do
Predictive analytics has transformed the landscape of agentic commerce by enabling businesses to anticipate customer needs before they materialize into conscious desires.
Machine learning algorithms accurately identify emerging trends and seasonal shifts by leveraging historical data, including purchase patterns and browsing behavior.
These sophisticated models enhance inventory management while improving customer satisfaction through timely, personalized recommendations.
Companies implementing these systems have witnessed conversion rate increases of up to 20%, as AI agents deliver relevant suggestions at prime moments.
This proactive approach streamlines operations and creates a seamless shopping experience, with products appearing just as customers realize they need them.
Operational Excellence Through AI-Powered Inventory Management
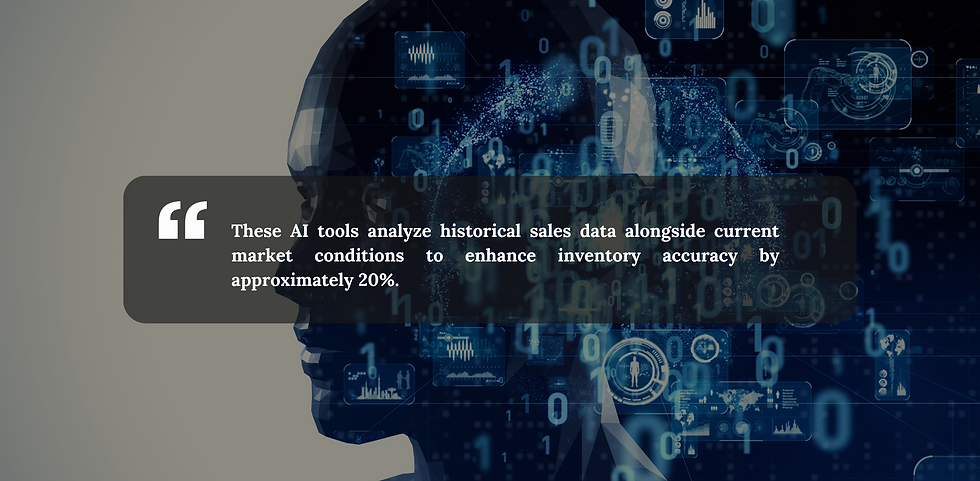
AI-powered inventory management represents the backbone of genuinely efficient agentic commerce systems. By leveraging machine learning algorithms, retailers can predict demand patterns with remarkable precision, reducing overstock and understock scenarios by up to 25%.
These AI tools analyze historical sales data alongside current market conditions to enhance inventory accuracy by approximately 20%.
Integrating machine learning into inventory management creates unprecedented operational efficiency, decreasing costs through waste reduction and optimized logistics.
Real-time tracking enables swift adaptation to fluctuating consumer demands, while automating routine tasks like stock replenishment frees valuable resources for strategic initiatives. This ultimately delivers seamless customer experiences through intelligent resource allocation.
Fraud Detection and Security: Protecting the Agentic Commerce Ecosystem
As digital transactions proliferate across agentic commerce platforms, sophisticated fraud detection systems powered by machine learning have become essential guardians of marketplace integrity.
ML algorithms analyze transaction patterns in real-time, identifying anomalies while reducing false positives by up to 50% compared to traditional methods.
Organizations implementing these technologies report 30% decreases in financial losses from fraud.
ML techniques like clustering and classification enable proactive risk mitigation by leveraging historical data to predict threats and adapt to evolving tactics.
This technological fortification protects the ecosystem and cultivates consumer trust, with 85% of shoppers preferring brands that deploy advanced security measures.
The Future Landscape: ML Applications That Will Define Next-Gen Shopping Experiences
The future scenery of agentic commerce extends beyond security concerns into transformative shopping experiences driven by sophisticated machine learning applications.
Advanced algorithms will enable hyper-personalized offers by analyzing consumer behavior in real-time, significantly increasing conversion rates. Predictive analytics will optimize inventory management, reducing stockouts by up to 30%.
Multi-agent collaborations across retail operations will streamline processes from sales to supply chain management, driving down costs. Meanwhile, automated complex interactions like subscription renewals will create frictionless customer experiences.
These innovations collectively represent a paradigm shift where machine learning becomes the cornerstone of next-generation commerce ecosystems.
How BSPK Clienteling Unified Commerce AI Can Help
BSPK Clienteling Unified Commerce AI transforms traditional retail interactions by bridging physical and digital shopping experiences.
By deploying sophisticated algorithms that analyze customer behavior across channels, BSPK enables retailers to deliver hyper-personalized recommendations and service.
The platform seamlessly integrates purchase history, browsing patterns, and customer preferences into actionable insights for sales associates.
This empowers frontline teams to anticipate needs, suggest complementary products, and maintain relationship continuity regardless of channel.
BSPK's architecture supports scalable implementation across enterprise systems while maintaining data security and privacy compliance, which are critical components for sustainable agentic commerce adoption in competitive retail landscapes.
Conclusion
Machine learning is the cornerstone of agentic commerce's evolution, seamlessly bridging customer desires with retail capabilities. As algorithms advance, the shopping ecosystem will increasingly anticipate needs, personalize experiences, and optimize operations with unprecedented precision.
This technological synergy doesn't merely enhance commercial transactions—it transforms them into intuitive, efficient interactions that benefit consumers and retailers alike.
Comentários